
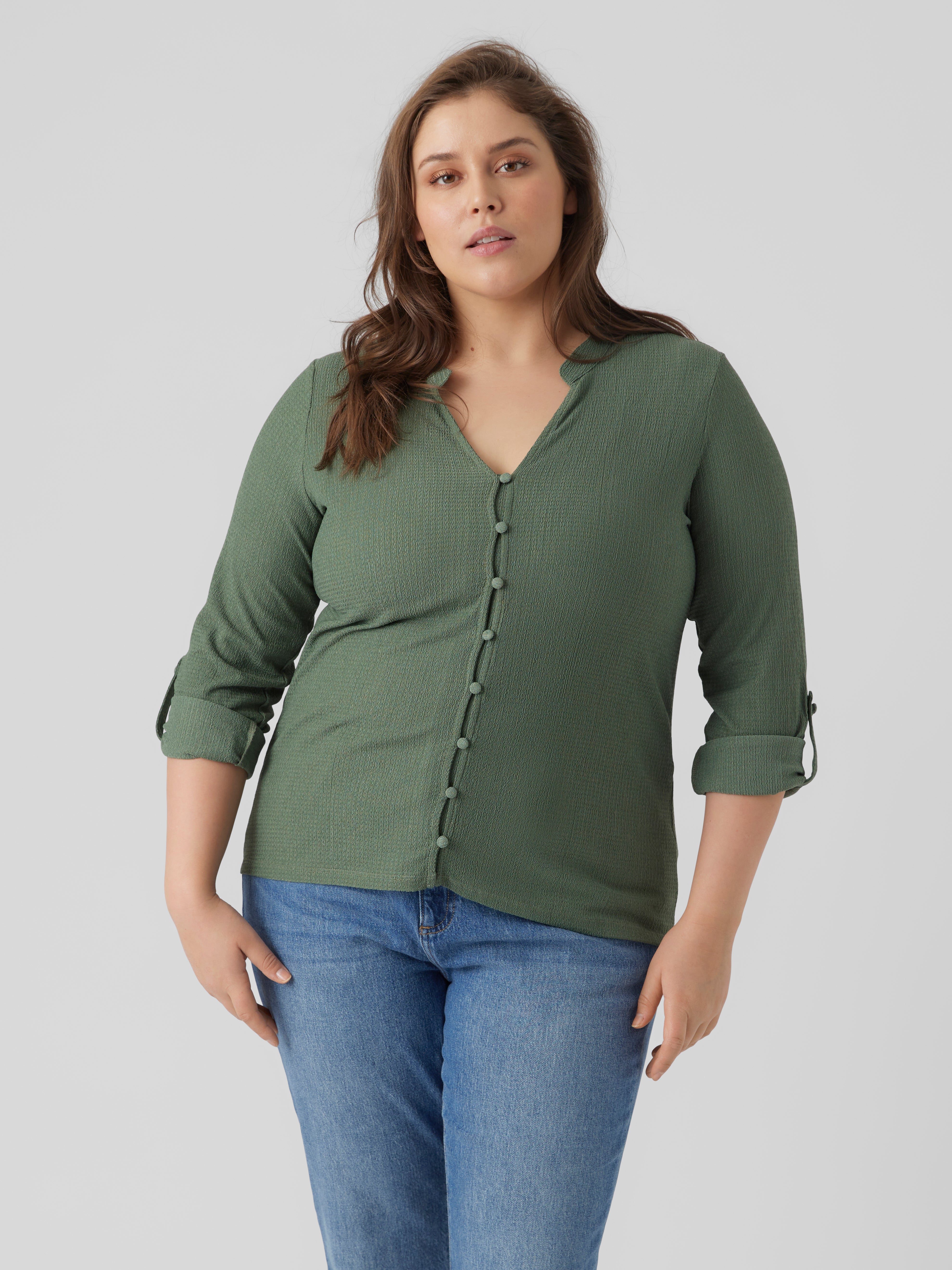
Fitting curves in PythonĪ great portion on this article was based on another blogpost, that was aimed at R users, and used R libraries with ready-made equations and built-in routines to facilitate the life of the data scientist. I also won’t go deeper into each model purpose and usage, as it’s not the point of the article.
Supertuxkart curve fit code#
In this article I’ll first present the main tools we’ll be using to fit the models, and then explain a series of useful nonlinear models + code + graph of the model for whenever you need to fit such marvelous equations. Log(y) = log(\beta_0) + x\cdot log(1 + \beta_1)Īnd then call $y'=log(y)$, $\beta_0’ = log(\beta_0)$, $\beta_1'=log(1 + \beta_1)$, you can re-write the Exponential Growth as:Īnd fit a OLS (Ordinary Least Squares) using this formula, as this is a linear model (this is called a log-linear model)! Finally, equation $(3)$ is a nonlinear model, as the regression is nonlinear in the parameters $\beta_0, \beta_1, \beta_2, \beta_3, \beta_4$ (I hope so, as I created this equation on the fly - if you find a linear parameterization for this equation please let me know). In this case we want to obtain the starting point and the growth rate At first you might think that $(2)$ is nonlinear, however you could manipulate the formula to obtain a linear parameterization: Equation $(2)$ is what we call an Exponential Growth formula, where usually $\beta_0$ represents the starting point, $x$ is a time measure and $\beta_1$ (usually called $r$) represents the growth rate. Y = \ \beta_0\cdot\sin\left(x^\right)\ +\ \beta_4Įquation $(1)$ is a simple line, and the parameters $\beta_0, \beta_1$ are linear on $y$, so this is an example of a linear model. To give more clarity about linear and nonlinear models, consider these examples: The model might not be linear in $x$, but it can still be linear in the parameters. So, for example, a quadratic equation $y = ax^2 + bx + c$ is linear, because this model is linear in the parameters $a, b, c$. It’s important to distinguish that when I say nonlinear I mean nonlinear in the parameters.

Describing these phenomena using mathematical equations give you the power to apply this constraint in the modelling phase: You just fit a function that has this monotonic property. For example if you’re modelling age x height of some insect it probably make sense that your height prediction should be monotonically increasing as age increases. Mathematical Properties: Sometimes it’s really important to guarantee some mathematical property on your model output.
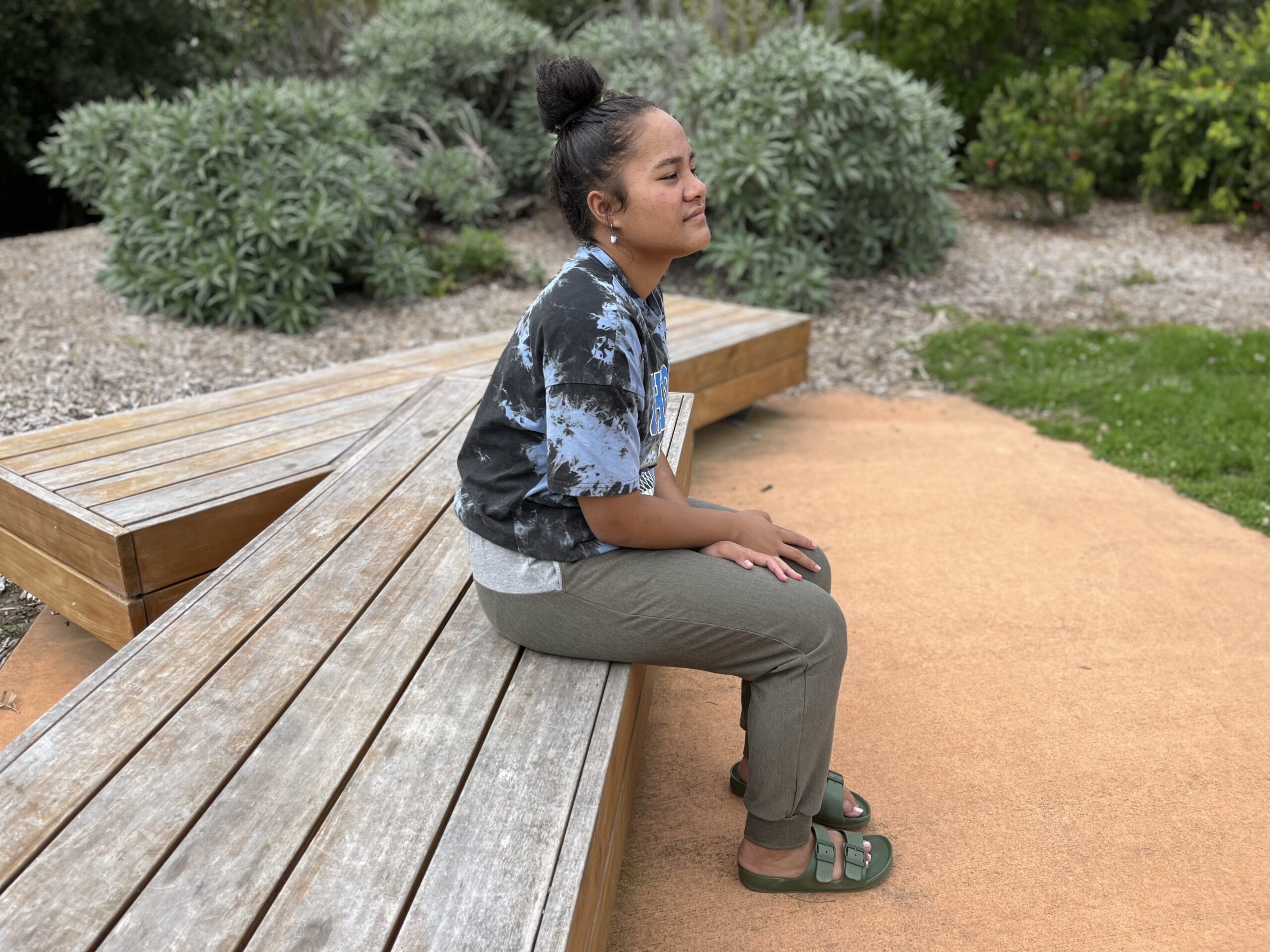
It’s much easier to explain what your model is doing if the output is interpretable. This will help not only the person that built the model in the future but also the business stakeholders. segment your data using the model, then fit interpretable curves on each segment). Important: this doesn’t mean you lose the power of your complex machine learning model, as you can still use the machine learning model alongside the curve you’re fitting (e.g. Interpretability: If you model an event using curves, your outcome is much more interpretable, since usually the mathematical equation has a meaning to each parameter. So, here are some considerations as to why would you use them: you want to model a phenomena using a curve that you can interpret later. It’s best to think about these nonlinear models in the context of curve fitting, i.e. They’re not necessarily a substitute for your complex (XGBoost, LightGBM, Random Forest, Neural Network, etc.) machine learning model. Now, before we get into the models, it’s important to note the context about why and when would you use these types of models. Since I tend to recurrently fit these models, t article will serve as a future reference for myself whenever I need to use them in the future, with easy to implement code (in Python). There are a lot of useful nonlinear models that guarantee useful mathematical properties and are also highly interpretable. However, not all problems can be solved with pure linear models. In Python you can achieve this using a bunch of libraries like scipy, scikit-learn, numpy, statsmodels, etc.

Fitting linear models is an easy task, we can use the least squares method and obtain the optimal parameters for our model. In statistics, we say that a regression is linear when it’s linear in the parameters. Asymptotic Model (constrained: starting from 0)Īll models are wrong, but some are useful.Asymptotic Model (Negative Exponential).
